Probabilistic Medicine
How ‘Big, Messy Data’ Can Guide Psychiatric Treatment
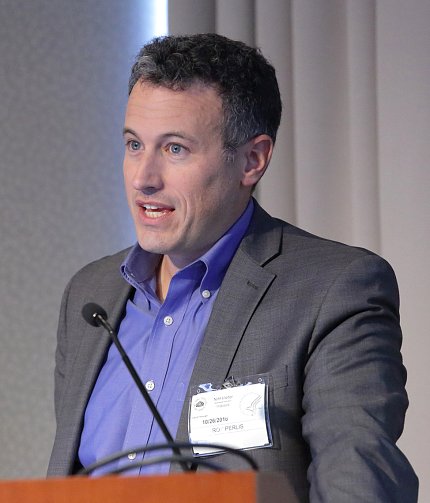
Photo: Ernie Branson
Psychiatry is an inexact science. Sometimes doctors must make educated guesses based on limited data. This scenario happened to Boston psychiatrist Dr. Roy Perlis, whose research focuses on treatment-resistant mood disorders. Frustrated after his lab’s large genomics studies failed to identify depression genes, Perlis began thinking outside the biomarker box.
“We’re always reasoning under conditions of uncertainty,” said Perlis, director, Center for Quantitative Health in Massachusetts General Hospital’s division of clinical research. “The genomics of psychiatric disease has turned out to be much harder than I think any one of us anticipated.”
Perlis, who also teaches psychiatry at Harvard Medical School, spoke at the NIMH Director’s Innovation Speaker Series at the Neuroscience Center on Oct. 26.
Faced with these challenges, Perlis and his collaborators wondered, “How do you get to personalized medicine when you can’t even find genes for a disease that affects 15-20 percent of the population?”
Beyond medicine, in recent years, personalization was plowing ahead in the tech world. Facebook launched targeted ads on news feeds based on collected data. Banks went a step further, acting on their trove of data by sending fraud alerts.
“The analogy in medicine would be, not only can we make guesses about how someone’s going to do over time, but also we need to be able to act on those predictions,” said Perlis. “It doesn’t do me any good if I can make a guess about something and not feed it back to the doctor or to the patient.”
So he started focusing on information they did have: electronic health records (EHRs), doctor’s medical notes, even simple surveys. “Six years ago, things were looking grim for genetic studies of depression. We couldn’t find genes; we didn’t know what we were going to do next,” said Perlis. “We had this crazy idea that we could map health systems using medical records and use that to build a biobank that would drive drug and biomarker discovery.”
First, his group partnered with 23andMe and Pfizer to use data from an online survey on depression history. Analyzing 75,000 cases of depression and 200,000 healthy controls, they were able to identify 15 novel loci for depression and additional targets and pathways that are still under study. “This was validation for me that big, messy data sets can still be useful,” he said.
They then ascertained that respondents with more risk variants associated with depression also tended to have more symptoms and comorbidities such as anxiety, insomnia and obesity, confirming findings from large epidemiological studies. “This is not a replacement for doing large-scale biobank studies or large-scale case control studies,” Perlis emphasized. “This is a complement to those kinds of studies.”
In addition to surveys, another important resource for personalized medicine can be EHRs, which include diagnostic codes, demographics, prescriptions and other relevant patient data. This accumulated information can help assess mental health risk and shape health interventions, such as medicines to add or remove from a patient’s regimen.

Photo: Ernie Branson
“As a field, we are conditioned to treat randomized controlled trials as our gold standard and they’re very good for certain things,” such as determining a drug’s effectiveness, Perlis said. “But if we’re going to develop more precise strategies for providing care for patients, we as a field of medicine need to get comfortable with using big, messy data sets,” or what he calls probabilistic medicine.
NIMH’s landmark STAR*D study treated 4,000 people with the antidepressant citalopram and then randomized treatments for patients who didn’t improve. “STAR*D made a major contribution to how we think about what to do when the first antidepressant doesn’t succeed,” Perlis said. "The question is, can we build on STAR*D without requiring another 5 years and tens of millions of dollars?"
To try to do so, his lab studied some 100,000 depression patients using only information from EHRs and learned that fewer than 10 percent who started antidepressants were ever prescribed an additional or different one. Currently, with collaborators at Harvard, his lab is trying to understand the factors that might guide decisions about next-step treatments for depression.
As a supplement to EHRs, his group also started looking at medical chart narratives— the notes written by doctors—to understand symptoms that might not appear in the diagnostic codes. They developed algorithms, such as natural language processing, to search and view the narratives in an automated way. “Our traditional diagnostic system in psychiatry doesn’t capture a lot of the variations that are probably important in understanding these diseases,” Perlis said.
When combining EHR data with notes from natural language processing, Perlis and his team found they could build better risk models. These approaches also allow them to map psychiatric symptoms across large clinical populations, understanding symptoms where diagnoses overlap and where they’re different.
“Now, all of a sudden, we can make predictions about who’s at high risk for bouncing back into the hospital after discharge,” he said. Doctors can then try a range of interventions such as a medication or occupational therapy for higher risk patients or a phone call or web-based follow-up for lower risk patients.
Before embarking on time-consuming, costly studies, said Perlis, this methodology lets them look across the health system to assess risk and possible low-cost interventions. They can then re-contact certain patients of interest and at that point spend time and money on targeted studies to learn more about these populations.
“We can make good predictions now and we’re getting better at it,” said Perlis. “I’m afraid we use our aspiration to identify biomarkers as an excuse not to use the immediately useful clinical data that we have.”
At Mass General, with NIMH and NHGRI support, they have also developed a cellular biobank where they collect samples, study drug responses, conduct cognitive and psychological assessments and link to EHRs to assess the full range of medical illness. They’re studying how brain cells develop over time and connect to each other. They can then test the effects of different medications to try to understand the cellular abnormalities associated with brain diseases.
“We’re reaching a point where we have a first set of genes; now we have a set of model systems we can use to understand what those genes are doing,” said Perlis. “We have a whole health system we can use to understand what those variations look like at a population level. The hope is that we can go back in and try to use that same resource to try to find better interventions, not just make predictions about our existing ones.”