Ask the Right Questions
Google Engineer Discusses Machine Learning in Medicine
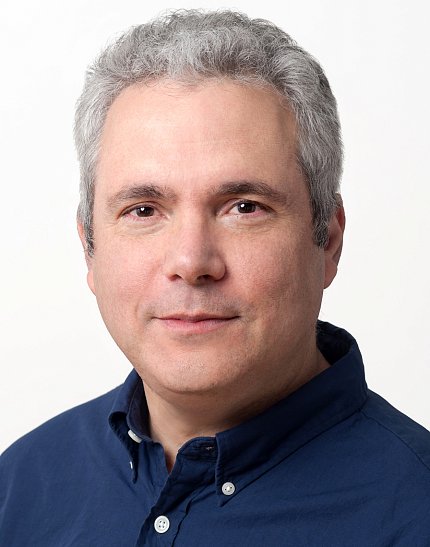
Not long ago, it was the stuff of science fiction. Today, it’s all around us: Our cell phones recognize images and speech, detect faces and auto-complete our sentences. Computers can learn to track customer habits to predict purchases and target advertising. They can also learn to detect fraud and deflect spam. Machine learning can interpret data for increasingly accurate predictions, and it has vast potential for biomedical applications.
A subset of artificial intelligence, machine-learning programs train computers to learn by example, explained Philip Nelson, director of engineering at Google Research, who spoke at a recent NIA artificial intelligence working group webinar. Such a program, Nelson said, showing a photo collage, could quickly discern the chihuahuas from the blueberry muffins. The magic is in the data, he said, which machine-learning programs use to look for patterns and correlations to make their own predictions.
“One of our hopes in applying machine learning in scientific contexts,” said Nelson, “is that it will discover these correlations and previously undiscovered patterns for humans to follow-up and understand what the root mechanisms might be, and to essentially propose new theories.”
One type of machine-learning model uses neural networks for complex, deep learning tasks. After a sluggish start, said Nelson, neural nets—circuitry that can learn distinguishing features from raw data and root out unwanted correlations—are getting sharper and faster with bigger models.
“If you give [the networks] a lot of data, they really start learning, quite impressively,” he explained, excited about breakthroughs during the past decade. “Neural nets needed the power of today’s computation hardware.”
Ever since the Deep Blue supercomputer beat chess grandmaster Garry Kasparov in the late 1990s, the best chess players have been programs written by many engineers over many years, noted Nelson. But now, a neural net-based system called AlphaZero, starting from just the rules of the game and learning by playing itself, is a far better player than the work of all those engineers. “These networks are starting to best programmers at their own jobs, too,” he said.
Nelson doesn’t see this technology replacing doctors, but rather assisting them in a wide range of medical applications. One exciting area is diagnostics. Google recently teamed up with physicians to apply its Inception image-recognition model to detect diabetic retinopathy (DR). The condition, which can cause vision loss, even blindness, in people with diabetes, is not symptomatic until later stages, making early detection critical.

“When we were able to get enough data and apply modern machine learning,” said Nelson, “the results were spectacular.”
The Google team designed and trained a neural network using 130,000 retinal images that were graded by 54 ophthalmologists over an 8-month period. In the ensuing clinical trial involving thousands of patients, the results of which were published in a Dec. 13, 2016, JAMA article, their algorithms had high sensitivity and specificity for detecting DR from these images.
“We were basically as good as a board-certified ophthalmologist in diagnosing the image,” said Nelson. Two years later, after further improvements in the training data, he reported, “We are now as good as a panel of retinal specialists.”
It turns out, their machine can also predict age, sex, blood pressure and refractive error from the images. “We can predict your risk of a significant cardiovascular event on par with the Framingham score just from retinal fundus photos, without seeing the rest of your labs,” he said. They’re even able to see anemia in the fundus. “These machines now are able to see things that humans have never seen before.”
Nelson also cautioned about the risks of both false-positives and false-negatives. For example, a third of diabetics will develop DR, so diabetics should have their eyes checked every year. But that can be expensive and inconvenient, and many people don’t even know they have diabetes. That’s why automated screenings, at pharmacies or even train stations, could be effective. But too many false-positives might overwhelm a health care system with otherwise healthy patients, he warned.

Photo: Credit Google
On the other hand, when neural networks assist a doctor reading a scan, studies have shown that false-negatives are more problematic. Doctors are good at dispensing with false-positives, but if the machine misses an issue, the doctor is likely to miss it too. People tend to trust computers too much, Nelson said. “We want our models to be as accurate as possible, and it’s also important to optimize the operating point and the user interface for the human workflow around any machine-learning-based system.”
Another emerging area for machine learning is pathology. Can machines emulate a cytotechnician, highlighting areas of concern for the doctor to check? Can machines analyze slides and recapitulate what pathologists would say? “One of the most exciting areas to me,” said Nelson, “is can we directly predict the outcomes or the therapies from the slide?”
Accuracy, though, depends on slide quality—whether the image is in focus. How likely is it, for example, that there is cancer in those pixels?
“If you were that patient, if this makes the difference between being treated properly or not, if you’re the 1 in 1,000, all this matters,” Nelson said. “We needed to build an image-quality model to run alongside our diagnostic models to help us understand the confidence in our predictions.”
Machine learning can also distinguish one image from another using a deep-learning technique called image regression. The machine is trained on pairs of correlated images, for example an unlabeled phase contrast microscopy image, and that same sample labeled with immunohistochemistry (IHC).
“The machine doesn’t understand biology,” Nelson said, “but if it can learn morphological patterns from the unlabeled image consistent with biological features shown by IHC, it can learn to predict those features in-silico without having to actually label the sample.”
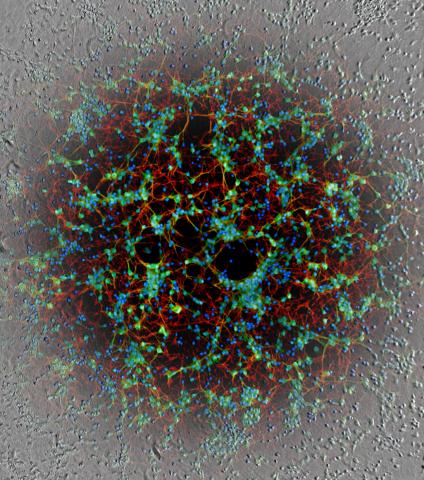
Photo: Credit Google
This can be especially useful in a pathology context, he added, where many simultaneous immune stains can be predicted while leaving the tissue otherwise unmodified, so it can be used for sequencing or other biochemical assays.
Another promising area for machine learning is phenotypic drug discovery, said Nelson. A neural network can be trained to predict an embedding, a small vector, that represents an image such that similar images produce vectors that are closer together. These embeddings can then be used to extract the visual variation seen in controls, to identify when a drug or treatment changes the cells and, most importantly, indicate which of these changes are alike.
In one experiment, researchers tested three drugs with the same mechanism of action. The embeddings for cells treated with these drugs clustered near each other but had three distinct sub-clusters because each drug had different secondary effects. What’s more, scientists could see the effects early.
“The images of the cells would move in embedding space very early, at very low doses,” Nelson said. Differences were then visible in that space when the dose increased. Many companies have begun using this technology.
The ability to morphologically cluster cells can give us new insights into the mechanisms of action of drugs, the effects of drugs in combination, and even treatments that might bring damaged cells back to a healthy state, explained Nelson. The hope for these technologies is to identify new patterns—new “Aha!” moments—that might lead scientists to new insights.
Machine-learning technology is already affecting health care, biology discovery and many other fields, he concluded.
“The potential is almost boundless, from imaging and diagnostics, to genomics, to wearable devices and other new biomarkers, to insights from medical records. These correlation engines might help us rethink longstanding biomedical challenges in the search for better outcomes. With machine learning, its eventual impact will be transformative. You have to start by asking the right questions.”