Redirecting Calculations
Mathematical Biologist Takes Up Covid Modeling
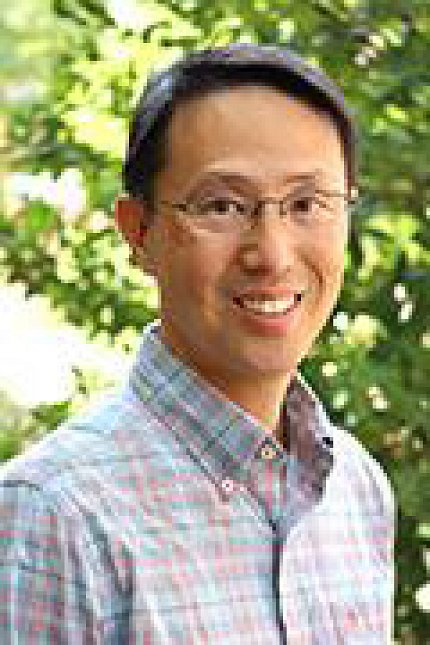
Like many NIH researchers faced with the devastating Covid-19 pandemic, Dr. Carson Chow shifted to mandatory telework, wondering how he could contribute to the world’s understanding of the virus. To many, though, his pivot to Covid research might seem incongruous.
A mathematical biologist, Chow is a senior investigator in NIDDK’s Laboratory of Biological Modeling. He has worked at NIH since 2004, using math to understand and model conditions such as obesity. Most recently, Chow has been employing mathematical modeling to develop a more complete understanding of DNA transcription. He acknowledges that it’s a “super-complicated process” with many steps that scientists do not yet have the tools to observe and comprehend.
“Biology consists of a lot of data—gathering data—and it’s a mostly experimental field,” he explains. “But sometimes you need to make sense of the data; it’s not immediately obvious what the data means, and that is where mathematical modeling can be helpful.” Chow uses his modeling in concert with molecular biologists to help piece together data that is accumulated from studying DNA transcription.
Models can augment data supplied by physical research. “The data gives you a snapshot of what’s going on, but it’s a snapshot in time,” Chow says. “You can fill in the gaps with a mathematical model.” The data can be used to inform a prediction, such as in modeling asymptomatic Covid cases.
He became interested in Covid modeling when NIH went virtual in March 2020. Suddenly relegated to his attic office for the foreseeable future, he began thinking about how he could contribute to the current understanding of Covid-19. He found himself drawn to asymptomatic cases, which no one knew much about at the time.
“It’s a computational question,” Chow says, “because at that moment people were taking data—the number of cases being reported—and the number of people that were dying from those cases, and the number of people recovering from cases. The question was, could you infer from that information the hidden number of people who are infected?”
The “hidden number” Chow referred to is the number of unreported cases, or people who are infected and shed the virus, but experience no or very mild symptoms. Current understanding is that roughly 1 out of every 5 Covid cases will be unreported.
Chow recruited several current and former fellows from his lab and got to work building models. They first had to familiarize themselves with the principles of epidemiology and the mathematical tools commonly used to understand disease spread. Then, he says, they “assembled some modern ideas from statistics,” and “tried to put together a model to see if [they] could infer what the hidden number of cases [was].”

Photo: Ben Chambers
The team employed an SIR-type model for the spread of disease, which represented possible states of the members of a population affected by an infectious disease (Susceptible, Infected, Case, Recovered, and, added in some models as SIR[D], Death). Health agencies report the number of cases, recoveries and deaths but the total number of susceptible and infected individuals are unknown and are predicted by the model.
Chow utilizes latent-variable Bayesian statistical methods in concert with the SIR models. Bayesian modeling uses probability to represent uncertainty within a model, which, in Chow’s research, can account for variables such as deaths unrelated to Covid, disease progression effects, population mixing and errors in classifying and reporting the data used in the research.
Using these models, he estimated that the number of unreported cases in April 2020 in the U.S. was 9 unreported for every 1 recorded. However, that number has dropped since.

Chow is currently collaborating with Dr. Kaitlyn Sadtler, another pivot scientist, in her work using antibody assays to detect asymptomatic Covid cases. Sadtler’s data helps inform Chow’s models, and Chow’s models can simulate a larger population than Sadtler could ever sample.
He attributes his breadth of projects to the flexibility of his field.
“There’s very little cost compared to an experimental lab,” he notes. “The only thing you lack in any pivot for a mathematician is knowledge.” He learns the science associated with whatever topic he wants to pursue, and then develops models based on the science he has learned.
“The only cost to a mathematician is opportunity cost,” he concludes.